Conditional distribution of the excesses
The second part of EVT is called the POT method (Peak Over Threshold) consists to use the observations that exceed a certain deterministic threshold and especially the differences between the observations and the threshold, called excess.
Let
a random variable with a CDF
and
which is a real enough large, called threshold. We define the excess over the threshold
the set of random variables
such as:

We look from the distribution
of
to define a conditional distribution
with respect to
for the random variable exceeding the threshold. We can define the conditional distribution of the excess such as:
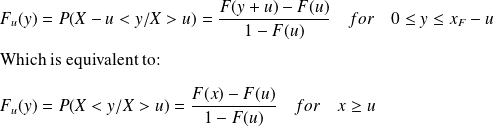
This method allows to determine by which PDF that we can fit the conditional distribution of excesses
when the threshold tends to the point
.
Lets
the conditional distribution of cumulative distribution function
with respect to a threshold
. When the threshold
tends to the value
:

The conditional distribution converges to the function
which corresponds to the Generalized Pareto cumulative distribution noted GPD. Generalized Pareto law is written as:

corresponds to Generalized Extreme Value law. For
(location parameter) the GPD law can be written as:
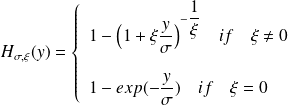